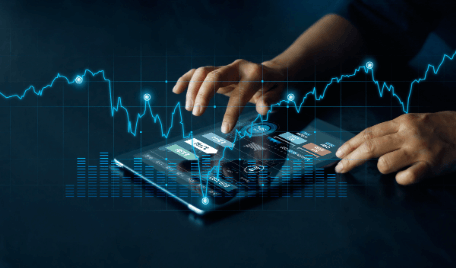
How are advancements in machine learning algorithms enhancing predictive analytics in sectors like finance and healthcare?
Predictive analytics is revolutionizing various industries by providing valuable insights that drive decision-making processes. Among these industries, finance and healthcare are particularly poised to benefit from advancements in machine learning (ML) algorithms. The combination of massive datasets and sophisticated ML techniques is enabling unprecedented levels of predictive accuracy and efficiency.
Introduction
The intersection of machine learning and predictive analytics is yielding transformative results across many sectors. Machine learning algorithms, with their ability to analyze vast amounts of data and uncover patterns, are at the forefront of this revolution. In sectors like finance and healthcare, where data is abundant and the stakes are high, the impact of these advancements is profound. This article delves into how modern ML algorithms are enhancing predictive analytics in these critical fields, improving outcomes, efficiency, and innovation.
The Role of Machine Learning in Predictive Analytics
Machine learning, a subset of artificial intelligence (AI), focuses on building systems that learn from data and improve their performance over time without being explicitly programmed. Predictive analytics leverages statistical algorithms and ML techniques to identify the likelihood of future outcomes based on historical data. The synergy between ML and predictive analytics is evident in their shared goal: making accurate predictions that inform decision-making.
Transforming Finance with Predictive Analytics
One of the primary applications of predictive analytics in finance is risk management. Financial institutions utilize ML algorithms to predict market trends, credit risks, and fraudulent activities. Advanced ML models, such as neural networks and ensemble methods, analyze historical transaction data to identify anomalies and predict potential risks. This proactive approach allows institutions to mitigate risks before they escalate, safeguarding assets and maintaining regulatory compliance.
Algorithmic Trading
Algorithmic trading, driven by ML, is revolutionizing financial markets. These algorithms analyze vast datasets at high speeds to identify trading opportunities and execute trades at optimal times. Predictive models help traders forecast stock prices, volatility, and other critical factors, enabling them to make informed decisions. This has led to increased market efficiency and reduced transaction costs.
Customer Insights and Personalization
Financial institutions are leveraging ML to gain deeper insights into customer behavior and preferences. Predictive analytics models analyze customer data to predict future needs, enabling personalized product offerings and services. For instance, banks can identify customers likely to benefit from specific financial products, enhancing customer satisfaction and loyalty.
Revolutionizing Healthcare with Predictive Analytics
In healthcare, predictive analytics is playing a crucial role in disease prediction and prevention. ML algorithms analyze patient data, including electronic health records (EHRs), genetic information, and lifestyle factors, to predict the likelihood of developing certain conditions. Early detection allows for timely interventions, improving patient outcomes and reducing healthcare costs.
Personalized medicine tailors treatment plans to individual patients based on their unique genetic makeup and health data. Predictive analytics models help identify which treatments are most likely to be effective for specific patients, minimizing trial-and-error approaches. This not only enhances treatment efficacy but also reduces adverse effects and healthcare expenditures.
Operational Efficiency
Healthcare providers are using predictive analytics to optimize operational efficiency. ML algorithms forecast patient admission rates, resource utilization, and staffing needs, allowing hospitals to allocate resources more effectively. This ensures that healthcare facilities are better prepared to meet patient demands, improving overall service quality.
Key Advancements in Machine Learning Algorithms
Deep learning, a subset of ML, involves neural networks with multiple layers that can learn complex patterns from large datasets. In finance, deep learning models are used for tasks like credit scoring and market prediction. In healthcare, they are employed for image analysis, such as detecting tumors in medical scans, and for predicting patient outcomes based on EHRs.
Natural Language Processing (NLP)
NLP algorithms enable machines to understand and interpret human language. In finance, NLP is used to analyze news articles, earnings calls, and social media sentiment to predict market movements. In healthcare, NLP helps extract valuable information from unstructured clinical notes, improving patient records and enabling better predictive models.
Reinforcement Learning
Reinforcement learning (RL) involves training models to make decisions by rewarding desired outcomes. In finance, RL algorithms optimize trading strategies by continuously learning from market conditions. In healthcare, RL can be used to develop personalized treatment plans by continuously adjusting to patient responses.
Challenges and Ethical Considerations
The effectiveness of predictive analytics relies heavily on the quality and availability of data. In finance, data from various sources must be integrated and cleaned to ensure accuracy. In healthcare, patient privacy concerns and fragmented data systems pose significant challenges. Ensuring data quality and overcoming these obstacles is crucial for reliable predictions.
Bias and Fairness
ML algorithms can inadvertently perpetuate biases present in training data, leading to unfair predictions. In finance, biased models might unfairly deny credit to certain groups. In healthcare, biased predictions could affect treatment recommendations. Developing algorithms that are fair and unbiased is essential for ethical and equitable outcomes.
Regulatory Compliance
Both finance and healthcare are highly regulated industries. Ensuring that predictive analytics models comply with regulatory requirements is essential. This includes data privacy laws, ethical standards, and industry-specific regulations. Compliance not only avoids legal repercussions but also builds trust with stakeholders.
The Future of Predictive Analytics in Finance and Healthcare
The future of predictive analytics lies in its integration with other emerging technologies such as AI and the Internet of Things (IoT). In finance, AI-powered predictive models combined with IoT data from connected devices can provide real-time insights into asset management. In healthcare, IoT devices can continuously monitor patient health, feeding data into predictive models for proactive care.
Enhanced Personalization
As ML algorithms continue to evolve, the level of personalization in predictive analytics will increase. In finance, this means more tailored financial advice and services. In healthcare, it translates to highly customized treatment plans that consider a wider range of patient-specific factors.
Real-Time Analytics
The ability to perform real-time predictive analytics will become more prevalent. In finance, real-time analysis of market data will enable faster and more accurate trading decisions. In healthcare, real-time monitoring and prediction of patient conditions will allow for immediate interventions, improving patient outcomes and reducing hospital readmissions.
Conclusion
Advancements in machine learning algorithms are significantly enhancing predictive analytics in finance and healthcare. These technologies enable more accurate predictions, better risk management, personalized services, and operational efficiency. However, challenges such as data quality, bias, and regulatory compliance must be addressed to fully realize their potential. As ML algorithms continue to evolve, the integration with AI and IoT will further revolutionize predictive analytics, leading to even greater innovations and improvements in these critical sectors.
FAQs
How do machine learning algorithms improve predictive accuracy in finance and healthcare? Machine learning algorithms analyze vast amounts of data to identify patterns and trends that traditional methods might miss. This leads to more accurate predictions and better decision-making in both finance and healthcare.
What role does deep learning play in predictive analytics? Deep learning models, with their ability to learn from complex and large datasets, enhance predictive analytics by providing more accurate and detailed predictions, especially in tasks like image recognition and natural language processing.
Can predictive analytics help in early disease detection? Yes, predictive analytics can analyze patient data to identify early signs of diseases, allowing for timely interventions and better patient outcomes.
What are the ethical considerations in using machine learning for predictive analytics? Ethical considerations include ensuring data privacy, avoiding biases in algorithms, and complying with regulatory standards to ensure fair and equitable outcomes.
How is reinforcement learning used in predictive analytics? Reinforcement learning is used to optimize decision-making processes by continuously learning from outcomes and adjusting strategies, particularly in areas like trading and personalized medicine.
What is the future of predictive analytics in healthcare? The future includes greater integration with AI and IoT, enhanced personalization of treatment plans, and the ability to perform real-time predictive analytics for immediate patient care interventions.